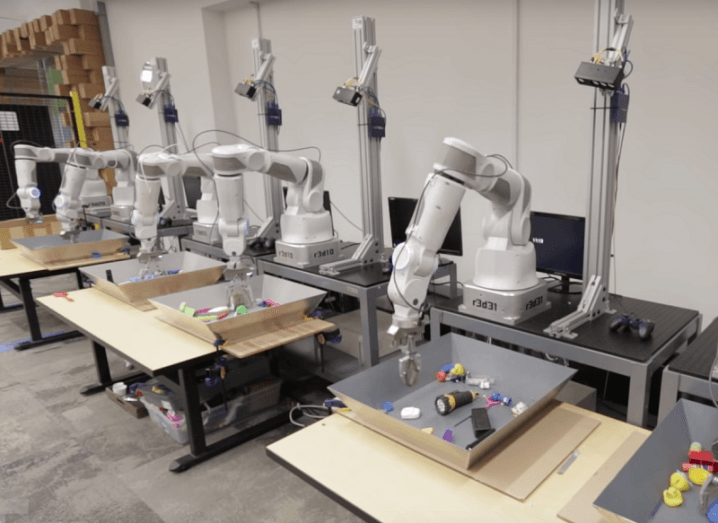
Image via YouTube
To make robot arms that are better and faster at picking up objects, Google’s robotics researchers have decided that the best course of action is to hook them all up to a singular neural network, or hive mind.
While dextrous robots with a hive mind sounds like something straight out of Terminator, the logic behind Google’s decision takes the simple approach of ‘two heads are better than one’ and expands this to 14.
Detailing the process in its development blog, Google’s electrical engineer Sergey Levine has published a paper on ArXiv about the developments the team has made in creating deep learning software that tries to mimic humans picking up objects.
Current code for dextrous robots tends to follow a familiar pattern of moving over a set of objects, scanning them, and then deciding which one it wants to pick up, referred to as the sense-plan-act paradigm.
As Levine points out in the blog post, humans and animals react rather differently than robots in that we tend to do very little planning when faced with a new object as our brains contain highly developed and intelligent feedback mechanisms about choosing an object.
“For example,” Levine says, “when serving a tennis ball, the player continually observes the ball and the racket, adjusting the motion of his hand so that they meet in the air.
“Can we train robots to reliably handle complex real-world situations by using similar feedback mechanisms to handle perturbations and correct mistakes?”
Picking up the pieces
That’s why Levine and his fellow researchers have decided that the best option is to hook up 14 robots to a hive mind – like the Borg race in Star Trek – and force them to pick up objects over and over again.
Once one of them figures out how to pick up a particular object, it will pass on the information to the others in the neural network.
Observing the behaviour of the arms over 800,000 grasp attempts, the researchers have shown no major improvement in terms of their ability to pick up objects in a more human-like manner, but their decisions in how they pick things up – such as where is the best place to grasp it – has reached almost human levels.
In what is a major step for their research so far, Levine and his fellow Google researchers are now looking to bringing the technology to a larger-scale.
“If we can bring the power of large-scale machine learning to robotic control,” he says, “perhaps we will come one step closer to solving fundamental problems in robotics and automation.”