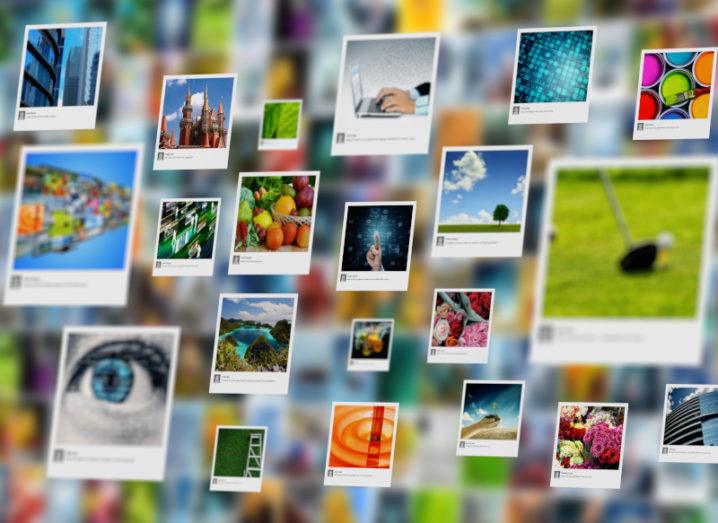
Image: © Nmedia/Stock.adobe.com
The study looked at some of the world’s most-cited machine learning datasets to investigate how error-ridden they are.
Datasets are a key part of training AI and machine learning systems. However, a new study has found widespread errors in some of the world’s most cited datasets, with an average error rate of more than 3pc.
For their study, a team led by computer scientists at MIT looked at 10 major datasets that have been cited more than 100,000 times, including ImageNet and Amazon’s reviews dataset.
Researchers found the number of errors in these datasets ranged from nearly 3,000 in one case (6pc) to more than 5m in another (10pc). The errors included mislabelled images, such as one breed of dog being confused for another and a baby being confused for a nipple.
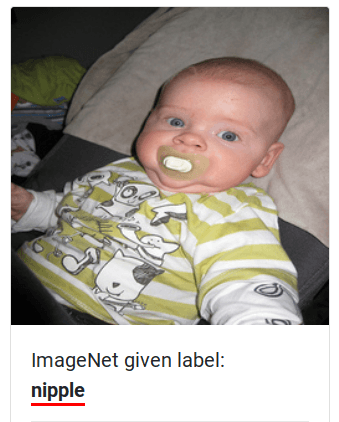
Image: labelerrors.com
While a certain number of errors can be expected, this becomes problematic when it comes to benchmark datasets that are highly cited, as error-ridden datasets could then inform other research or algorithms.
“We use ImageNet and CIFAR-10 as case studies to understand the consequences of label errors in test sets on benchmark stability. While there are numerous erroneous labels in the ImageNet validation set, we find that relative rankings of models in benchmarks are unaffected after removing or correcting these label errors,” the study said.
“However, we find that these benchmark results are unstable. Higher-capacity models (like NasNet) undesirably reflect the distribution of systematic label errors in their predictions to a far greater degree than models with fewer parameters (like ResNet-18), and this effect increases with the prevalence of mislabelled test data.”
Labelling errors could lead scientists to draw incorrect conclusions about which models perform best in the real world.
The researchers found that larger models are able to generalise better to the given ‘noisy’ labels in the test data, but said this is problematic because these models produce worse predictions than their lower-capacity counterparts when evaluated on the corrected labels for mislabelled test examples.
“In real-world settings with high proportions of erroneously labelled data, lower capacity models may be practically more useful than their higher capacity counterparts,” the study added.
The researchers said that while machine learning practitioners often choose which model to deploy based on test accuracy, these findings advise caution here, proposing that judging models over correctly labelled test sets may be more useful.
“It is imperative to be cognisant of the distinction between corrected versus original test accuracy, and to follow dataset curation practices that maximise high-quality test labels, even if budget constraints limit you to lower-quality training labels.”
Issues in much-cited datasets were highlighted last year by Abeba Birhane of University College Dublin, who helped uncover how the ‘80 Million Tiny Images’ dataset may have contaminated AI systems with racist, misogynistic and other slurs. The database of 80m images was developed by researchers at MIT, who withdrew the dataset.