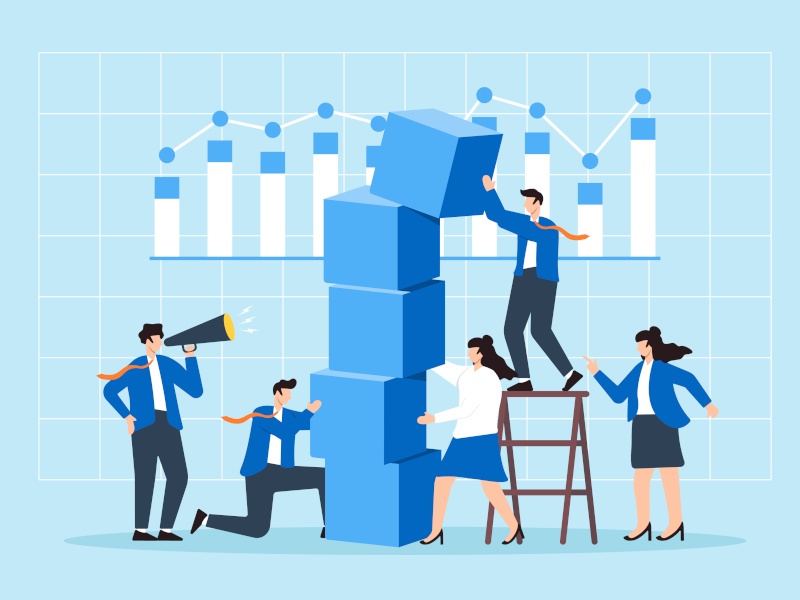
Image: © VZ_Art/Stock.adobe.com
For Luís Filipe Gonçalves, data and AI expert at Noesis, a strong foundation is the key to successful AI implementation, so take the time to get it right, he advises.
From predictive analytics to advanced data visualisation, artificial intelligence (AI) promises to revolutionise how businesses process and leverage information. Yet, before we charge headlong into an AI-driven future, there’s a critical question we need to ask: Are we truly ready?
The tools we have at our disposal, such as Qlik, Power BI and Tableau, offer incredible capabilities in data analytics. But are our organisations with all the systems, infrastructure and people prepared for the leap into AI? And even more for the generative AI rush?
AI hype v reality
AI is often sold as the ultimate solution to all data challenges. From automation to real-time insights, the promise of AI is vast. But implementing AI in data analytics isn’t as simple as flicking a switch.
Many companies rush into AI initiatives without a proper foundation, resulting in frustrations, missed opportunities and underwhelming results. Without a real data-driven vision, AI will be another idea, not a business decision tool.
Before adopting AI, businesses must first take a hard look at the state of their data ecosystems. AI thrives on vast amounts of clean, structured data, but how prepared are most companies in managing this data flow? Without the right groundwork, AI can’t deliver its full potential.
Know the present before changing the future
Dat visualisation tools such as Qlik, Power BI and Tableau have already changed how we interact with data. They help businesses visualise trends, drill down into specifics and make informed decisions quickly. These platforms offer an essential foundation for any AI initiative, but their full potential can only be realised if businesses address underlying data challenges.
Having the capability to explain the past is key to foreseeing the future with AI. It is mandatory to have this capability inside our organisations because, if we don’t know our past, we will not be able to look into the future. And we can only understand our past business decisions, our past goals and targets if we have tools that can support that data mining.
There are some vital areas where readiness is essential.
Data infrastructure
Before diving into AI, companies must evaluate their existing data infrastructure to ensure it is scalable and flexible enough to integrate advanced AI algorithms. AI relies heavily on fast and reliable access to data. If your infrastructure can’t support large-scale, real-time data flow, AI will struggle to deliver value. Scalability is the key driver for the organisation’s data infrastructure.
Data quality
‘Garbage in, garbage out’ is a phrase often used in the data world. AI needs clean, high-quality data to function effectively. While Qlik and Power BI have strong data-cleansing tools, the responsibility for ensuring data integrity ultimately falls on the business. If the data is incomplete, inconsistent or outdated, even the most sophisticated AI system will yield flawed insights.
Integration capabilities
The existing tools work best when integrated with other systems across the enterprise. This raises the question: Can your current ecosystem handle such integrations seamlessly?
AI can amplify insights when connected to CRM systems, marketing platforms and other enterprise software, but the integration capabilities of your current set-up need to be robust enough to support this interaction.
A non-siloed strategy is mandatory. If AI is another set of tools, another application disconnected from the ecosystem, AI will never be a business-as-usual strategy. It will be just another tool.
Talent and people
Even with the best tools in hand, the human element remains crucial. AI adoption requires data literacy across the organisation. Reskilling and upskilling are necessary to ensure your people can navigate this transition smoothly. Organisations can have the best strategy, the best set of tools, a well-defined framework, but without people with training and talent, an organisation will not take the correct advantage from this data-driven vision.
Collaboration, not replacement
AI is not here to replace existing tools, it’s here to enhance them. These platforms have evolved to incorporate AI features, such as predictive analytics and natural language processing, but this doesn’t mean they become fully autonomous.
Organisations should view AI as an enhancement to human decision-making. AI can process vast datasets quickly, but human intuition and strategic thinking will always play a pivotal role. In fact, many experts argue that AI works best when combined with human intelligence, particularly in complex, context-specific decisions. The last call should be always done by business owners, not by algorithms or generative AI outputs.
What if you’re not ready for full AI?
While the benefits of AI are clear, not every business is immediately ready to implement it at full scale. But here’s the trick: you don’t need to advertise that you’re not AI-ready. You can embark on ‘pre-AI’ initiatives without the world knowing you’re still preparing for the full leap into AI.
Understanding the analytical maturity curve of the organisation is a business differentiator. If we know where we are we can define where we were and how we want to proceed as an organisation. Doing this kind of assessment can map the organisation’s status quo and show hidden capabilities already in place across the organisation.
Launch an AI-FTF (AI Foundation Task Force). This isn’t a pre-AI project, it’s a strategic initiative that focuses on building the data, infrastructure and skills necessary for a successful AI-driven future.
Other initiatives that you can use freely include:
AI-DRP (AI Data Readiness Programme)
This focuses on cleaning, structuring and optimising your data so that when AI is fully deployed, the transition is smooth and effective.
AI-SIP (AI Systems Integration Plan)
This ensures that your existing platforms can seamlessly integrate with AI tools and algorithms, preparing for a future where these systems work hand in hand.
AI-TSP (AI Talent Skilling Programme)
This is designed to elevate the skills of your team, ensuring they can maximise the potential of AI once it’s deployed, and helping them understand how AI will reshape their roles and responsibilities.
By focusing on these initiatives, your organisation can make real, impactful progress toward an AI future without compromising current operations. These are not just preparation projects, they are critical steps in building the foundation for AI success in the future.
Is your business AI-ready?
While AI is undoubtedly the future of data analytics, it’s not a race to be first, it’s about being prepared. By ensuring that your data, infrastructure and people are ready, businesses can make the most of AI without the pitfalls of poor implementation.
Qlik, Power BI and Tableau will continue to play a crucial role in bridging today’s analytics needs with tomorrow’s AI-driven innovations. But, just as with any technological leap, success lies in preparation. Before you embrace AI, look back and ensure that your foundation is solid.
The future may be exciting, but readiness will always be key. Ensure your organisation is really at the forefront of AI transformation, whether today or tomorrow.
Luís Filipe Gonçalves is director of data analytics and AI at Noesis, an international tech consulting company. He has nearly 20 years’ experience in data analytics, business intelligence, QlikView and SAS. Currently, he leads a team of more than 100 professionals, driving data-driven and AI-powered innovations at Noesis.
Don’t miss out on the knowledge you need to succeed. Sign up for the Daily Brief, Silicon Republic’s digest of need-to-know sci-tech news.