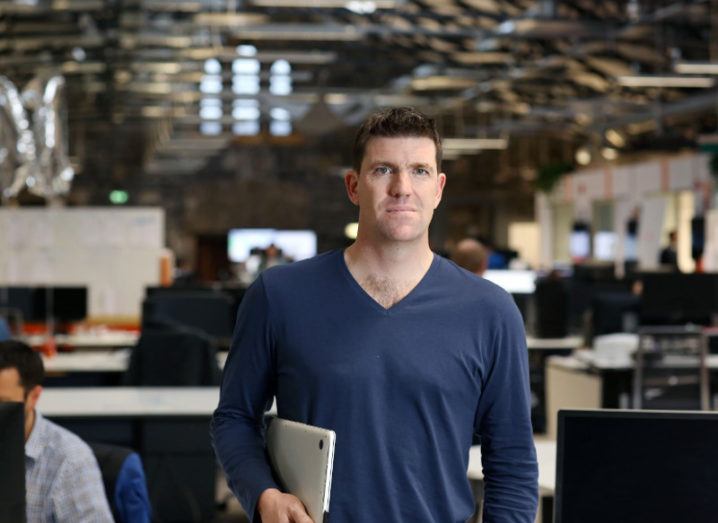
Graham O'Sullivan, Zalando. Image: Justin Mac Innes/Mac Innes Photography
The future of online retail will be data-driven, says Zalando’s head of engineering.
Graham O’Sullivan is head of engineering at Zalando in Dublin. Prior to joining Zalando, O’Sullivan was in charge of architecture and software at Unum.
Zalando is a major online fashion retailer. The Berlin-based company has a presence in 15 European countries selling various types of clothing to around 15m customers.
‘Essentially what you have here is Maslow’s hierarchy of needs for data science. We have a very deep mix of infrastructure engineering and data science’
– GRAHAM O’SULLIVAN
Zalando came to Dublin in 2015 with plans to create 300 jobs over a three-year period at its Fashion Insights Centre, a research-oriented hub that was set up to build data-driven products with data science and deep learning at their core.
O’Sullivan will be speaking next Thursday (28 September) at a Singlepoint breakfast on cloud-first machine learning at The Irish Aviation Authority Conference Centre in Dublin.
How would you describe your role at Zalando?
I fulfil two roles at Zalando right now. I am head of engineering and the dedicated owner of two platforms: Fashion Insights and Customer Data.
Zalando has internally reorganised in order to optimise for end-to-end delivery. That’s a very fancy way of saying Zalando has tried to minimise horizontal organisational structures and ensure that tech, commercial, product and operations are all aligned with one group with one owner. They call that owner a dedicated owner, or manager or decision-maker. So essentially I am the dedicated owner for the fashion content platform and the customer data platform for both of those.
My background is almost exclusively in engineering but as a dedicated owner you are expected to develop competencies across product and commercial. You are also expected to organise your part of the organisation to be able to deliver for the customer for whom you are ultimately responsible.
The origin myth of Zalando is that it began as three guys in a bedroom selling flip-flops online. But now it seems its product is data?
You are articulating possibly a future state or aspirational future state. Zalando is a huge operation – you go to Berlin and move around the offices, visit the warehouses and it’s incredible how big and how complex the operation is.
They have €3.2bn in net sales, 200m visits a month. They have 250,000 different articles of clothing they sell on the site. At heart, Zalando is still e-commerce. That’s the lifeblood. But it has really transformed into being a data-driven organisation with technology running in its veins.
Ultimately, the position it wants to achieve in the future is to be a platform player.
It wants to really figure out how to monetise and sell the data and the platform capabilities to other players in the European e-commerce ecosystem.
How deeply does data go into the Zalando organisation?
We have a fairly strong remit in Dublin to be involved in most aspects of data.
Some of the work we are doing with respect to data science is relatively standard. But, essentially what you have here is Maslow’s hierarchy of needs for data science. We have a very deep mix of infrastructure engineering and data science.
For example, we look to leveraging the use of all sources of data internally in the company – that includes data lake. But we also look to acquire data externally as well.
There are also well-understood ways of acquiring data externally and more novel ways of doing that. From an engineering perspective, we have explored both.
What we do then is we deploy a lot of our data scientists with engineering competencies in small focused teams to extract knowledge out of that data.
How engaged are you with AI, machine learning and deep learning?
We are engaged in deep learning, natural-language processing and, by and large, we are really expending quite a bit of effort. At least a third of our office consists of research-oriented PhD data scientists all looking to solve digital problems across a range of media – not just data, but different media types.
Ultimately, what we are looking to try to do is ensure that we can provide analytics at a base level, but also provide much deeper insights in order to influence some of the buyer cycle (this can be in some of the ways we purchase) and even to influence trends and potentially figure out what we may want to do in the future with respect to trend-spotters and buying.
We really see ourselves, in Dublin in particular, as platform providers, but we look to build out pretty coarse-grained APIs that can be used across a load of verticals. That means that we have to be fairly solid in our offering. We are building out foundational capabilities that we can stitch together relatively easily to provide new functionality in order to give value back to the business.
What platforms do you use? Is it all based in the cloud?
It is all cloud-based. AWS is our base layer and we use a lot of their services.
In terms of what we are doing from a data science perspective, it is a mix of bespoke data science models and some open-source and off-the-shelf. We are generally using Spark and we are using Kafka in relatively novel ways. It is then mostly all delivered through Restful API. We are also internally looking at streaming microservices.
It is very much cloud-first. We have completely gone away from internal data centres and internal infrastructure. There are some legacy systems but they are being migrated to the cloud.
How do you structure teams with data at their core?
It’s a very strong link between the organisational unit of the team and ensuring that the team is accountable. If they build it, they run it. And the only way you can ask a team to be fully accountable is if they control the entire infrastructure.
It generates efficiencies if teams are able to stand up their own stack, if they are responsible for monitoring it in run-time, if they are responsible for ensuring that it can scale elastically, and they can do all of that within the team unit themselves. It allows them to innovate much more quickly.
In general, the cloud-first mentality is one we have really bought into and is the core part of our DNA.
What are the big trends you foresee for data in the future?
Deep learning is solving problems that were previously considered unsolvable and it is solving them increasingly more quickly. Cloud providers are also making things like GPUs, building in TensorFlow etc. All of these things are becoming more ubiquitous and easier to handle.
We think that soon a lot of deep learning and data science will become a lot more commoditised and it would be easier for companies who are feeling left behind to rapidly get a foothold in the market and be able to leverage deep learning and data science techniques to be able to do things. That’s one trend.
Deep learning is going to solve problems that were intractable in the past. We are investing more and more in deep learning.
From a retail perspective, people want to be inspired, and once people are on our site we are okay. But people are looking for new and novel ways to be inspired with respect to fashion, and this opens up whole new avenues for technologies to get engaged and involved in.
Some of the things we would think about would be how to engage people outside our core properties, be able to understand behaviours better and be able to present them what they want, personalise around what they want, and ensure we are not just doing simple recommendations, but a much more nuanced and subtle view.
All of the technical capabilities that allow you to be able to do that are beginning to become more easy to construct and to build.
Want stories like this and more direct to your inbox? Sign up for Tech Trends, Silicon Republic’s weekly digest of need-to-know tech news.