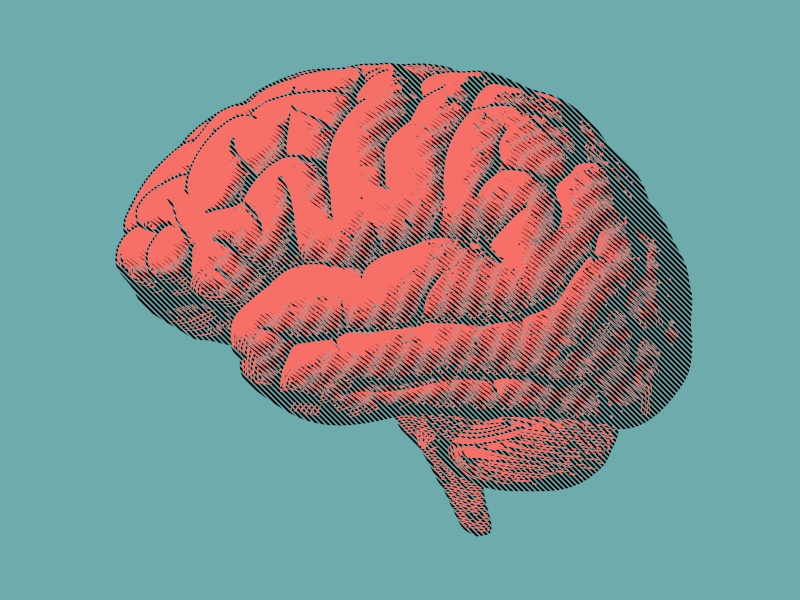
Image: © jolygon/Stock.adobe.com
What if AI could provide doctors with a second opinion? Expert in medical physics, Dr Andreas Stadlbauer believes it could soon be a possibility.
Prof Andreas Stadlbauer develops biomedical imaging techniques to create 3D images of brain tumours to support clinical diagnostics. His recent research identifies machine learning methods to quickly and accurately diagnose primary brain tumours, which will help in developing personalised treatments for patients.
Stadlbauer studied technical physics at the Vienna University of Technology and during his studies completed research at CERN in Switzerland. With the support of his supervisor Prof Ewald Moser, he completed a PhD in the imaging of brain tumours as part of a cooperation between Friedrich-Alexander University Erlangen-Nuremberg and the Vienna University of Technology.
He studies brain tumours from a radiological perspective and from a neurological perspective, which he says gives him a “broad insight” into the topic. He works across the Institute of Diagnostic and Interventional Radiology at St Pölten University Hospital and Karl Landsteiner University of Health Sciences, Austria.
For Stadlbauer, the value of engaging wide audiences in his work is “the resulting discourse with patients, colleagues and the media, which leads to an interesting exchange of ideas and new insights”.
Here, he tells us more about his current research.
Tell us your current research.
My research focuses on biomedical imaging and image processing. In this context, I am working on the energy metabolism of the brain and its pathologies. I have been working intensively with artificial intelligence (AI) and deep learning in medical imaging for several years now. In the clinical field, we work with proven and established AI applications and currently still mainly with 2D images. The technology is developing rapidly. This makes it difficult to keep track, but we have a lot of ideas. Before an innovation can be used in routine diagnostics, it must be sufficiently established. We have to be able to understand how the results are achieved. I am convinced that AI applications will support doctors as a ‘second opinion’ in decision-making in the near future.

Image: Andreas Stadlbauer
I am currently working on a project supported by Karl Landsteiner University to develop deep-learning algorithms for medical image analysis and integration into clinical diagnostics to create 3D images that can be used by experts on the tumour board for decision-making.
In your opinion, why is your research important?
Today, we can generate anatomical, physiological and metabolic images of tumours in 3D. Metabolic images relate to oxygen consumption and physiological images allow conclusions to be drawn about the tumour’s vascular system. There is a lot of potential in physiological and metabolic images; they provide us with important information earlier than anatomical images. If it were possible to combine the information content of different 3D images from different points in time, this would bring enormous progress, especially in the diagnosis of recurrence after surgical tumour resection and radiochemotherapy. So-called generative deep learning algorithms could also generate images that show us what a tumour would look like in three months’ time, for example. We are talking about huge and complex volumes of data here. We are not yet able to process these volumes of data sufficiently well. Generative AI like this would be a huge step forward and highly relevant in clinical terms.
What inspired you to become a researcher?
The desire to work permanently in research only really came to me during my doctoral thesis. So it wasn’t a childhood dream of mine to become a scientist, although my interest and fascination for nature had been awakened in me at an early age. Even as a physics student, I always intended to go into business after my doctorate. It wasn’t until the exciting research on metabolic imaging of brain tumours that I slipped into science and have been fascinated by it ever since. I can’t remember a specific moment, but the work and publication of my first paper was definitely a formative phase.
What are some of the biggest challenges or misconceptions you face as a researcher in your field?
One of the biggest challenges is that biological processes cannot be predicted with 100pc certainty. For a physicist who is used to describing nature using exact, mathematically formulated laws, this is a real challenge at first. This is all the more true, for example, when assessing the effects of therapy on tumour diseases. As a physicist, you have to learn to live with these uncertainties, especially since they often affect life-determining questions for the patients affected.
Do you think public engagement with science and data has changed in recent years?
Science and scientific findings have certainly become more of a focus of public interest in recent years. However, little is known to the general public about how scientific findings are created. Unfortunately, this opens up a lot of room for pseudoscientific claims and even ‘alternative facts’ that are believed without question by some people because they fit their worldview. Social media then acts as a multiplier for the misinformation.
Find out how emerging tech trends are transforming tomorrow with our new podcast, Future Human: The Series. Listen now on Spotify, on Apple or wherever you get your podcasts.