
Image: © BlueBeans/Stock.adobe.com
TechWatch editor Emily McDaid reports from the latest 4IRC debate, discussing the role of ethics in the world of machines.
On 16 October, a Fourth Industrial Revolution Challenge (4IRC) meet-up on deep learning was held to discuss the ethical implications of AI.
Getting together in the new Catalyst Belfast Fintech Hub, contributors attended from corporate, start-up and academic worlds. Host Emer Maguire introduced the first of four speakers.
Speaker: Brian McDermott, full-stack developer at Allstate
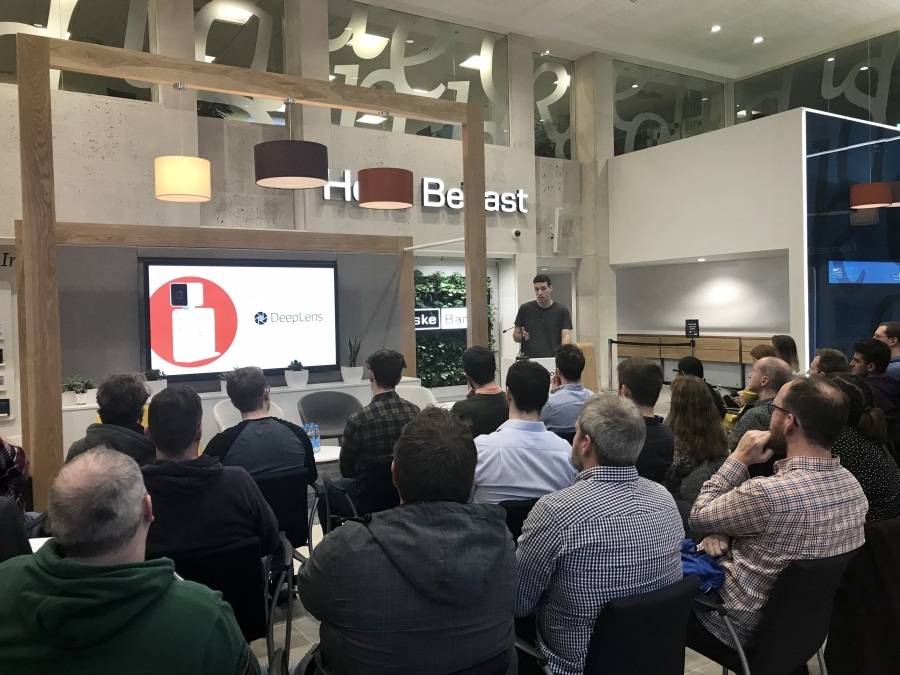
Brian McDermott. Image: TechWatch
McDermott focused on a practical introduction to machine learning (ML) techniques.
“This will be a stats-free presentation. I’ll show you how to get started in machine learning and deep neural networks.
“There are three different types of ML: supervised, unsupervised and reinforcement.”
Examples:
- Teachable Machine with Google.com: You can train a machine to recognise three visual inputs and play a different sound for each – this is supervised.
- Unsupervised: An app that asks you to draw a saxophone in 20 seconds. It keeps guessing what the object is as you draw.
- Google DeepMind’s Deep Q-Learning: You can teach this app to play and win basic games.
- Lyrebird: Create your own voice avatar – it learns to speak just like you.
“This is quite promising technology, but someone unethical could do a lot with this. In terms of ethical questions, Amazon’s hiring tool, based on AI, was biased towards hiring men. This demonstrates that AI is only as good as the data put into it.
“The best online resources to learn about AI are Google AI, and the online course, machine learning by Andrew Ng on Coursera. This course is free or if you want to get a certificate, you can pay for that – he’s the best of the best.”
Speaker: Padraic Sheerin, co-founder of Squad
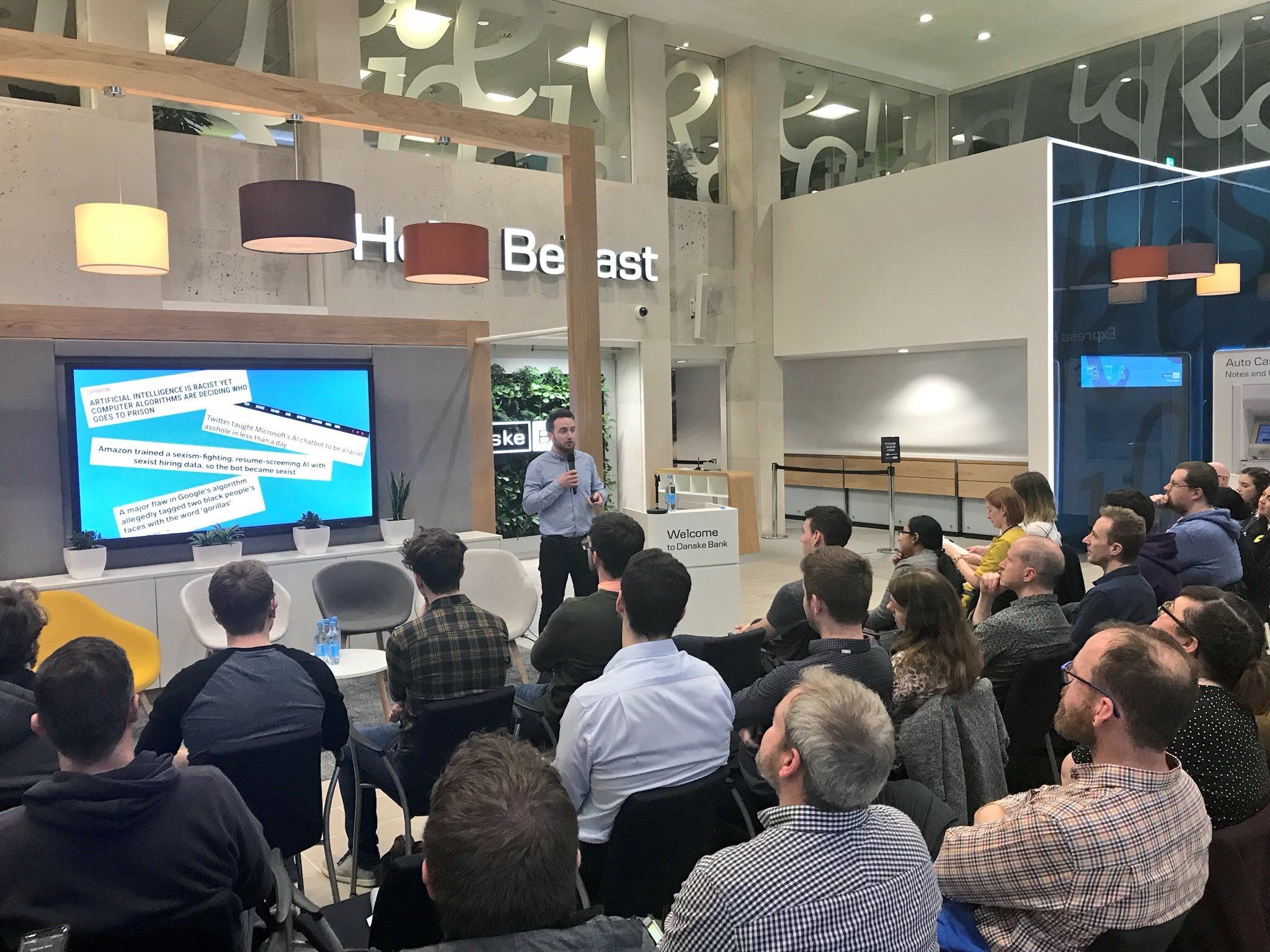
Padraic Sheerin. Image: TechWatch
Sheerin’s fintech start-up, Squad, helps young people to save their money.
“I want to share a view that I’ve been thinking about: how can AI achieve more for society than MLK [Martin Luther King] did?
“Let me start with my time working in the US insurance industry. Actuaries set insurance prices for years based on a pooled risk – pooling people together, then adjust price to drive consumer demand.
“We used machine learning to come up with a better estimate of a customer’s demand based on how likely they are to buy something at a certain price. We asked, could I figure out someone’s price sensitivity using a model? It predicted how likely we were to shop around. We could make prices 10 to 20pc lower than the risk-adjusted prices.
“We adjusted the price of millions of people’s policies – the company was doing great, the stock price was outperforming the market. This was a perfect example of using AI to drive a really good business outcome. But before long, the regulators came in, and they had a problem with it.
“At the time we thought it was really unfair, but what I’ve learned since is that by taking AI and replacing a human decision, you use a level of detail that’s never been seen before in human history. For the first time in history, regulators could look at how you weighted people – now it could see whether you’re biased or not. I can measure that bias and I can decide if it’s right or wrong.
“They ended up banning the practice in many states. There was a general fear of AI and they didn’t understand the models. As a result of that ban, people ended up paying more for insurance.
‘AI is only as good as the data put into it’
– BRIAN MCDERMOTT
“This is to illustrate the challenges we have with AI in future. Human bias: the trolley problem. A self-driving car will have to decide which of two people to knock down. Decisions are hard regardless of who’s making the decision. Even as flawed as humans’ morals are, what other baseline could we use to code our machines? There is no other baseline to be used.
“What’s important to understand is that even though AI runs the risk of encoding bias into an algorithm that exists for a long time, we now have a way to measure that bias that was never there before.
“I ask you this: would Amazon be as quick to change its hiring process had the AI not shown up the bias? I don’t think so – not that quickly.
“AI shines a light and helps us understand. Do you start to slow down AI? You can’t do that for three key reasons:
- Globalisation
- Massive benefits it can give the developing world
- Force to drive equality
“MLK helped us overcome known bias. AI can shine a light on unconscious bias to help us eradicate that, and that kind of bias is even worse.”
Speaker: Angelina Villikudathil, researcher at Ulster University
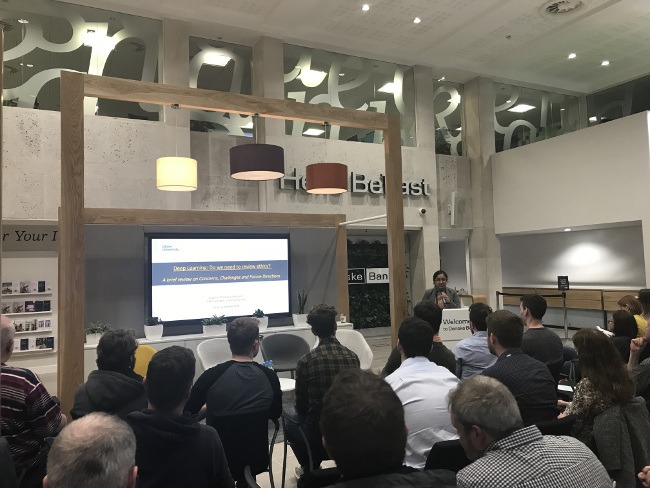
Angelina Villikudathil. Image: TechWatch
“Humans, not robots, are responsible agents. Robots need to explain themselves – how did you do what you did, and why?”
Papers she recommends reading:
- Machine Bias, ProPublica (2016)
- Concrete Problems in AI Safety
“In terms of fairness, how we can make sure ML systems don’t discriminate? Ensuring ML doesn’t impinge on human rights, prioritising wellbeing, accountability (establish responsibility and avoid potential harm), transparency (addressing the concepts of traceability), technology misuse and awareness (hacking, misuse of data, exploitation).”
Angelina Villikudathil is currently using AI techniques to stratify patients with type 2 diabetes. Her data-driven study is looking at the disease pathway for diabetes, especially through co-morbidity. Co-morbidity refers to when a patient suffers from two related conditions – for instance, diabetes plus heart disease.
‘If we employ artificial neural networks, the network relearns from existing predictions. This is similar to how the human brain works’
– ANGELINA VILLIKUDATHIL
In a separate interview, she said: “We identify biomarkers – proteins, genes, anything that stratifies patients. By determining the molecular phenotype and developing a range of biomarkers, we can assess individual patients.”
Her project applies AI algorithms to look at responders and non-responders to a particular drug. “Different patient groups respond to the treatments, and others don’t. Analysing this data with machine learning gives us a greater understanding of the disease itself.”
A major benefit to this study is that doctors can diagnose a disease earlier. More tailored therapies could be developed for patient sub-groups. Also, the research is providing clinical insight into particular genes.
Why is it important to use AI techniques? Villikudathil said: “If we employ artificial neural networks, the network relearns from existing predictions. This is similar to how the human brain works, the ability to continually learn from past experiences.”
The patients involved in Villikudathil’s study have all been treated at Altnagelvin Hospital in Co Derry. Around 254 patients have consented for their data to be used in the study – SNP genotypic data. In addition to the aim of reducing diagnosis times for patients, this could also save the NHS “billions of pounds”, said Villikudathil. She pointed to this recent BBC article questioning whether AI could save the NHS.
At the completion of the project, Villikudathil will earn her PhD in machine learning for biology applications. I ask her whether she’ll stay in Northern Ireland. “There are opportunities worldwide,” she said. “I can see many options – I’m just waiting for the right one.”
Speaker: Pete Wilson, management consultant for VeroZen
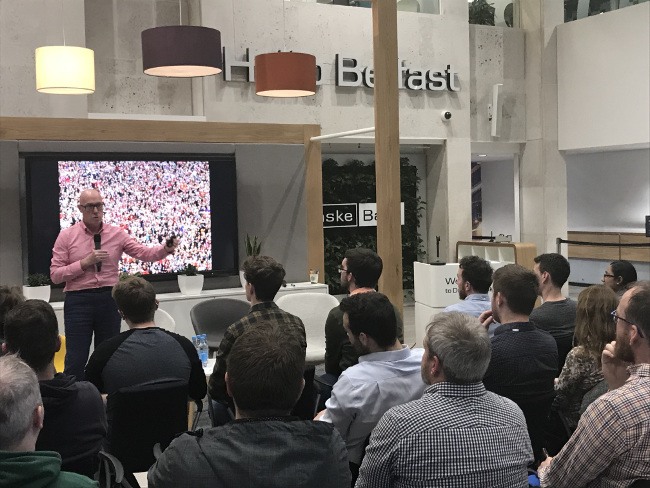
Pete Wilson. Image: TechWatch
“Padraic raised a really interesting story about the trolley problem. It’s interesting that there’s cultural influence on how humans answer the question.
“Now, we’re in a position where we have emergent tech but haven’t got framework.
“I recommend Klaus Schwab’s book – it says we all have the capability to inform the future.
“I’d suggest that our ethics are permanently under review, especially now with the likes of Twitter … a cesspool of opinion.”
Panel session
Questions from the audience were read out by Maguire.
Maguire: “Padraic, what’s the difference between deep learning and machine learning?”
Sheerin: “Deep learning learns relationships with the data that a human could never recognise. It trains a neural network and finds a level of patterns we can’t see.”
Maguire: “How can you teach an AI machine something as complex as ethics?”
Wilson: “For example, in GDPR’s rules it states that you can’t allow a computer to make a decision that you can’t explain as a human.”
Villikudathil: “That is why we’re breaking down each decision that’s being made. We can enforce ethics.”
Wilson: “There’s a workforce time bomb in many countries. If they don’t automate, their economy is going to tank.”
Maguire: “To avoid bias, can you just remove that data – for instance, gender and race data?”
Sheerin: “The short answer is yes. You can train an AI system using artificial data, and the question is: how do you get that data to look fair?”
Maguire: “How is AI used in climate change and food waste?”
Villikudathil: “I can give a general answer. I know you can build predictive models over time. Using daily weather patterns, it can learn a pattern.”
McDermott: “Models can take the usage from different grocery stores based on sales. By better predicting the demand, you can better meet the demand with the right displayed food.”
Maguire: “What went wrong with Microsoft?”
Sheerin: “A chatbot created comments on issues of the day. Twitter already had a lot of bots that were already on there. If Microsoft’s chatbot learned from those bots because there were more bots than people (bots were created to amplify negative comments), it reflected those comments.”
The next 4IRC debate, Digital Identity: Inclusive or Invasive?, will be held on 1 November at Queen’s University Belfast.
By Emily McDaid, editor, TechWatch
This article was formed from two articles that appeared on TechWatch; one on the 4IRC event recap and one focused on Angelina Villikudathil’s research