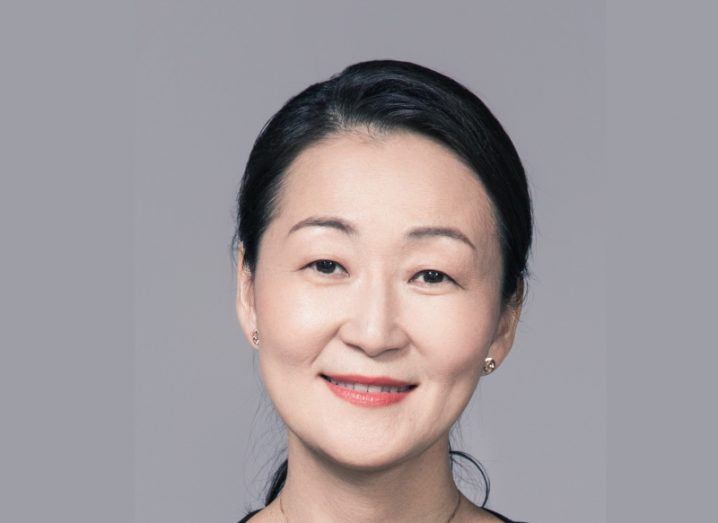
Dr Feiyu Xu. Image: SAP
AI expert Dr Feiyu Xu talks about the different approaches to AI globally and how natural language processing has changed throughout her career.
A big part of automation is the use of machine learning and artificial intelligence. However, the ways in which these technologies are deployed depend on many external factors, from funding and investment to regulations and location.
Dr Feiyu Xu, the global head of AI at German software giant SAP, has a unique view on this due to her background.
Having grown up in China, Xu completed her undergraduate, master’s degree and doctoral studies in artificial intelligence in Germany. She then began her career as a scientist and worked for many years in artificial intelligence research.
She worked at the German research centre for AI (DFKI) and co-founded and managed an AI start-up before moving into industry.
“First I went to Lenovo, which took me back to my home country, China,” she told SiliconRepublic.com.
“My recent stay in China made me realise how strongly China embraces AI because the need for automation and intelligence in their civil infrastructure is so urgent. In a country with 1.4bn inhabitants, innovation – in particular, big data and AI technologies – is needed to improve the standard of life and work.”
Xu has gained a keen insight into how AI is being used across the world and said there are currently at least three approaches to AI globally.
As mentioned, she said the Chinese or Asian way tends to be very open to the use of big data and AI and the state invests massively in digital solutions. “In particular, the commercialisation of AI applications has been very successful.”
In the US, Xu said AI innovation is led by large corporations and enabled by their investments. “The US is leading the AI technology research and AI applications.”
Finally, the European approach is often focused on regulation and safe-guarding before innovation, and “public opinion is still rather sceptical about digital transformation, AI and big data”.
“Europe has been very successful in basic research and also has a long tradition in AI research. But when it comes to commercialising AI, European industry has fallen behind the US and China, especially in AI for the internet and consumer products.”
Xu said this is clear from the book AI Superpowers by Kai-Fu Lee, where the author sees China and the US as the superpowers, while Europe isn’t even a close third place.
‘The stricter regulations [in Europe] force us to develop rules and methods to deal with the challenges’
– DR FEIYU XU
A 2020 Deloitte study found that in Germany, companies favour buying off-the-shelf AI rather than developing the tech themselves. But Xu said there is a realistic chance for Germany to become a leader in the international AI race if it capitalises on its ability to develop AI, especially in the enterprise software arena.
“For Europe, I see increased opportunities in the field of business AI, such as enterprise AI, industrial robotics, health AI and smart manufacturing.”
This is not the first time Europe has been called out for lagging behind other nations in this space. Earlier this year, a report from the European Parliament’s special committee on artificial intelligence in a digital age said that the EU had “fallen behind” in the global tech leadership race.
“We neither take the lead in development, research or investment in AI,” the committee stated. “If we do not set clear standards for the human-centred approach to AI that is based on our core European ethical standards and democratic values, they will be determined elsewhere.”
The lag in innovation is believed to be partially due to the focus on AI regulations in the EU. In April 2021, the European Commission proposed new standards to regulate AI in a bid to create what it calls “trustworthy AI”. These proposals seek to classify different AI applications depending on their level of risk and implement varying degrees of restrictions.
However, Xu said that while the legal frameworks in Europe “seem very strict”, there are ways the EU can turn this into an advantage.
“The stricter regulations force us to develop rules and methods to deal with the challenges. The GDPR and the emerging AI regulations require the explainability and transparency of AI solutions that contribute to decision-making,” she said.
“On the one hand, they pose more hurdles for AI development. Thus, they urge AI research and development to invest more effort in trustworthy AI.”
How natural language processing has changed
A major area of Xu’s expertise lies in natural language processing (NPL), which is a computer program’s ability to understand human language, whether it be written or spoken. In 2013, she won a Google Focused Research Award for her contribution in the field of NLP.
Xu said the pace at which NPL has advanced in recent years is “truly unprecedented”, with many problems that were previously deemed unsolvable having since been solved.
“Looking at recent high-profile results like PaLM in which pre-trained models explain common sense reasoning (and explain why jokes are funny), or DALL-E generating images from textual descriptions, the boundaries have yet to be established,” she added.
“I am most excited about the fact that these advances also have a major impact on business AI, as many of the advances are about getting more done but with less data – and access to data is always an obstacle to applying AI in the enterprise.”
‘With each leap, NLP is producing better results with fewer data points’
– FEIYU XU
Xu said that at the start of her research career, working in NLP meant applying a variety of means, ranging from rule-based methods for basic tasks to statistical measures and graph algorithms, all the way to traditional machine learning.
“Each problem was addressed by a specific combination of these methods, and each NLP researcher needed a deep understanding of each of those to develop solutions,” she explained.
“With the advent of deep learning methods, NLP solutions started to look more similar. Early on, deep learning was considered yet another tool in the box, but as it significantly increased accuracy on many tasks, it was used more and more.”
These advances then led to the emergence of transformer-based pre-trained language models such as BERT and GPT-2. These models were trained on a vast amount of text by trying to complete sentences or fill in blanks, and the focus for solving NLP tasks switched from methods to data.
“The most recent leap, where bigger and bigger models – based on the same transformer components as BERT – are trained on more and more data, enables these models [such as] GPT-3 to address NLP tasks without even fine-tuning,” she said. “The models auto-complete the next examples by simple pattern matching, with surprisingly sophisticated and usable results.
“With each leap, NLP is becoming easier to apply to new tasks, requiring less knowledge and producing better results with fewer data points.”
Beyond NLP, Xu said there are two AI trends she sees having a major impact in the future: the integration of information extracted from texts and from structured sources such as databases; and the explainability of black-box machine learning.
She said the information integration will “enable the explicit representation of knowledge and enable machines and humans to work on structured knowledge jointly”, which will be crucial for business AI where “correctness is paramount”.
In terms of black-box machine learning, she said transparency will be key to the success of business AI.
“When enterprise users work with machine learning-based recommendations or predictions, users need to understand how they came to be able to judge if they can be trusted to identify errors and mistakes,” she said.
“With transparency then, machine learning methods can simplify the lives of enterprise users, allowing them to get their work done more quickly, and plan their businesses with greater foresight.”
10 things you need to know direct to your inbox every weekday. Sign up for the Daily Brief, Silicon Republic’s digest of essential sci-tech news.