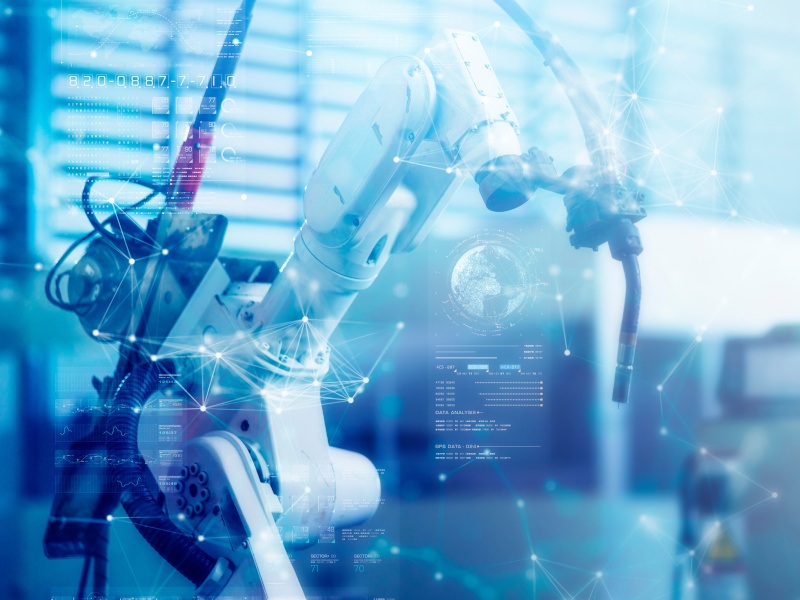
Image: © DIgilife/Stock.adobe.com
UL’s Dr Muftah Fraifer says the interdisciplinary work of autonomous systems research is ‘a constant balancing act’.
Dr Muftah Fraifer describes the team he works with at University of Limerick and the Lero Research Centre for Software as “fantastic”.
“I am fortunate to collaborate with a highly diverse team of researchers from across the globe.”
Fraifer is a postdoctoral researcher in the areas of AI and autonomous systems and a self-described “internet-of-things enthusiast”.
He has undergraduate and MSc degrees in electronic engineering, and he completed a PhD in 2018 in Limerick. He has authored 13 articles and works as a reviewer for several international journals.
“Ultimately, what drives me as a researcher,” Fraifer says, “is the opportunity to make tangible contributions to society by developing innovative technologies that push the boundaries of what’s possible, improve lives and solve pressing challenges in various sectors.”
Tell us about your current research.
My current research focuses on blended autonomy vehicles as part of my role at Lero. I am particularly interested in integrating networking, IoT and computer vision to address practical challenges in autonomous systems and machine vision applications.
I am involved in projects such as developing systems to detect and classify different load types in warehouse environments using models like YOLO, Mask R-CNN and others. My work extends beyond detection to include the precise measurement of load dimensions, ensuring adequate spacing and preventing collisions.
Through my research, I aim to advance AI-driven autonomy by bridging theoretical models with real-world applications in industrial and vehicular systems.
In your opinion, why is your research important?
My research is important because it addresses real-world challenges in automation, autonomy and industrial efficiency, particularly in environments where precision and safety are critical. By integrating machine vision models, such as YOLOv8 and DETR, with IoT technologies, I develop systems that enhance operational accuracy, reduce human intervention and improve decision-making in complex scenarios.
For example, in warehouse environments, my work on load detection and measurement helps ensure safe spacing and reduces the risk of accidents or material damage. This is vital for improving supply chain efficiency and meeting the increasing demands of modern logistics.
Similarly, my contributions to blended autonomy vehicles advance the development of smarter transportation systems, making autonomous operations safer and more reliable.
Ultimately, my research contributes to advancing Industry 4.0 goals, enabling smarter, interconnected systems that support safer, more efficient and sustainable operations across various domains.
What inspired you to become a researcher?
My inspiration to become a researcher stemmed from a deep fascination with how technology can solve real-world problems and improve the way we live and work. Growing up, I was always curious about the potential of machines and systems to think, learn and adapt. This curiosity led me to pursue an academic path where I could blend my passion for problem-solving with my interest in technology.
The pivotal moment came during my doctoral studies, where I had the opportunity to work on projects that combined computer vision, machine learning, and IoT and IxD fields that I found both intellectually stimulating and practically impactful.
As I advanced in my research, I realised the transformative power of blending different domains – like computer vision and networking – to create smarter, safer and more efficient systems. The potential to contribute to the development of autonomous vehicles, smart warehouses and IoT-driven solutions was a major motivator for me.
What are some of the biggest challenges or misconceptions you face as a researcher in your field?
One of the primary challenges is obtaining high-quality, diverse datasets. For tasks such as object detection and load measurement in warehouses, reliable and accurately labelled data are essential. However, real-world environments are often dynamic and unpredictable, necessitating careful curation and annotation of datasets – a time-consuming and resource-intensive process.
Another significant challenge is integrating multimodal technologies. Combining machine vision models, such as YOLO, Mask R-CNN, and DETR, with other algorithms, sensors and autonomous vehicle systems presents unique difficulties. These technologies operate on different time scales, utilise varied data types and have distinct processing requirements. Ensuring seamless communication and real-time decision-making between them requires not only technical expertise but also innovative approaches to system design.
The need for real-time data processing is another major hurdle. Autonomous systems must operate quickly and accurately, whether detecting a load on a shelf or ensuring the safety of a vehicle. Achieving this requires optimising models for efficiency without compromising performance, which is particularly challenging when working with large datasets or complex algorithms.
Furthermore, AI models trained in controlled environments often struggle to perform under real-world conditions. Variability in lighting, background noise and physical interference in settings like warehouses can lead to underperformance. Addressing this requires continuous testing, fine-tuning and robust model validation.
Lastly, the interdisciplinary nature of autonomous systems, involving fields such as robotics, networking and control systems, adds another layer of complexity. Ensuring that all these components work harmoniously while advancing the state of the art is a constant balancing act that requires effective collaboration and innovative solutions.
Do you think public engagement with science and data has changed in recent years?
Public engagement with science and data has significantly evolved in recent years, particularly during the Covid-19 pandemic. As an AI researcher in machine vision, I’ve observed how data visualisations, predictive models and real-time dashboards became essential tools for public understanding and decision-making. Concepts like ‘flattening the curve’ and vaccine efficacy brought scientific data into everyday conversations, fostering awareness but also exposing gaps in data literacy. The pandemic highlighted both the power of data-driven solutions and the challenges of misinformation, emphasising the need for transparent communication and accessible tools to bridge the gap between complex science and public understanding.
Don’t miss out on the knowledge you need to succeed. Sign up for the Daily Brief, Silicon Republic’s digest of need-to-know sci-tech news.