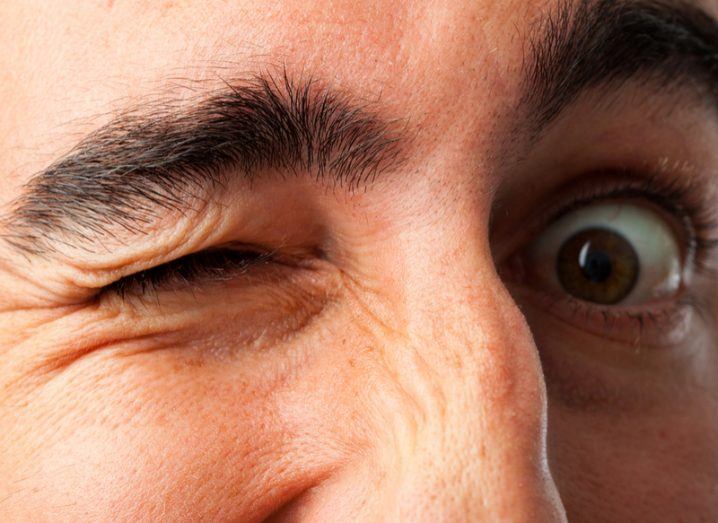
Image: Kues/Shutterstock
University at Albany’s Dr Siwei Lyu has discovered the crucial giveaway that will help you tell a deepfake video from the real deal.

A version of this article was originally published by The Conversation (CC BY-ND 4.0)
A new form of misinformation is poised to spread through online communities as the 2018 US midterm election campaigns heat up. Called ‘deepfakes’ after the pseudonymous Reddit account that popularised the technique – which may have chosen its name because the process uses a technical method called ‘deep learning’ – these fake videos look very realistic.
So far, people have used deepfake videos in pornography and satire to make it appear that famous people are doing things they wouldn’t normally. But it’s almost certain deepfakes will appear during the campaign season, purporting to depict candidates saying things or going places the real candidate wouldn’t.
Because these techniques are so new, people are having trouble telling the difference between real videos and the deepfake videos. My work with my colleague Ming-Ching Chang and our PhD student Yuezun Li has found a way to reliably tell real videos from deepfake videos. It’s not a permanent solution, because technology will improve. But it’s a start, and offers hope that computers will be able to help people tell truth from fiction.
What is a deepfake video?
Making a deepfake video is a lot like translating between languages. Services such as Google Translate use machine learning – computer analysis of tens of thousands of texts in multiple languages – to detect word-use patterns that they use to create the translation.
Deepfake algorithms work the same way. They use a type of machine-learning system called a deep neural network to examine the facial movements of one person. Then they synthesise images of another person’s face making analogous movements. Doing so effectively creates a video of the target person appearing to do or say the things the source person did.
Before they can work properly, deep neural networks need a lot of source information, such as photos of the persons being the source or target of impersonation. The more images used to train a deepfake algorithm, the more realistic the digital impersonation will be.
The eyes have it
There are still flaws in this new type of algorithm. One of them has to do with how the simulated faces blink – or don’t.
Healthy adult humans blink somewhere between every two and 10 seconds, and a single blink takes between one-tenth and four-tenths of a second. That’s what would be normal to see in a video of a person talking. But it’s not what happens in many deepfake videos.
When a deepfake algorithm is trained on face images of a person, it’s dependent on the photos that are available on the internet that can be used as training data. Even for people who are photographed often, few images are available online showing their eyes closed. Not only are photos like that rare – because people’s eyes are open most of the time – but photographers don’t usually publish images where the main subjects’ eyes are shut.
Without training images of people blinking, deepfake algorithms are less likely to create faces that blink normally. When we calculate the overall rate of blinking and compared that with the natural range, we found that characters in deepfake videos blink a lot less frequently in comparison with real people. Our research uses machine learning to examine eye opening and closing in videos.
This gives us an inspiration to detect deepfake videos. Subsequently, we developed a method to detect when the person in the video blinks. To be more specific, it scans each frame of a video in question, detects the faces in it and then locates the eyes automatically. It then utilises another deep neural network to determine if the detected eye is open or closed, using the eye’s appearance, geometric features and movement.
We know that our work is taking advantage of a flaw in the sort of data available to train deepfake algorithms. To avoid falling prey to a similar flaw, we have trained our system on a large library of images of both open and closed eyes. This method seems to work well and, as a result, we’ve achieved a more than 95pc detection rate.
This isn’t the final word on detecting deepfakes, of course. The technology is improving rapidly, and the competition between generating and detecting fake videos is analogous to a chess game. In particular, blinking can be added to deepfake videos by including face images with closed eyes or using video sequences for training.
People who want to confuse the public will get better at making false videos – and we and others in the technology community will need to continue to find ways to detect them.
By Dr Siwei Lyu
Dr Siwei Lyu is associate professor of computer science and director of the Computer Vision and Machine Learning Lab at the University at Albany, State University of New York.